UAV avoid obstacles technology
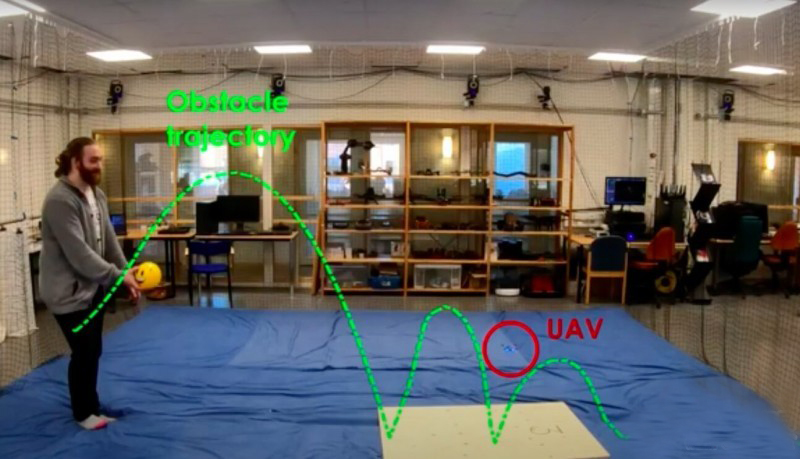
Autonomous unmanned aerial vehicles (UAVs) have shown great potential in a wide range of applications, including automatic package delivery and surveillance of large geographic areas. However, in order to complete tasks in the real environment, drones need to be able to effectively navigate and avoid obstacles in the surrounding environment.
Researchers at Luleå University of Technology in Sweden and the California Institute of Technology have recently developed a calculation technology based on nonlinear model predictive control (NMPC), which can provide UAVs with better navigation and obstacle avoidance capabilities. The NMPC method they used was published in a paper in IEEE Robotics and Automation Letters. The method is based on the structure of OpEn (optimization engine), which is a parameter optimization software developed by Dr. Pantelis Sopasakis, Queen’s University Belfast .
One of the researchers who conducted this research, Björn Lindqvist, told TechXplore: “Our team has previously published several works on automatic obstacle avoidance and navigation of drones.” “In our recent one. In our research, we began to use NMPC to extend the concept of obstacle avoidance to include direct consideration of moving or dynamic obstacles. Our goal is to provide a technical demonstration of how modern intelligent control structures make unmanned aerial vehicles a common use in urban environments, for example In an environment where the surrounding environment is always moving, and avoiding collisions is very important to ensure the safety of people and other vehicles.
NMPC is an optimized solution that can be used to solve various practical problems. This solution can predict the future state of the system based on the mathematical model of the system and a series of control inputs acting on the system at a given time.
The forecast covers multiple future time steps, and these time steps are collectively called the "forecast range." The solution then calculates control inputs in a way that allows the system to most effectively accomplish a set of desired goals (for example, reference tracking). , Avoid obstacles, keep constraints, etc.).
Lindqvist and colleagues show in the paper that NMPC-based models can provide advanced autonomous navigation and obstacle avoidance functions for drones in changing environments (such as cities). More specifically, they use the NMPC algorithm to predict the trajectory of obstacles in the environment around the drone, while also using a classification model to distinguish different types of trajectories and predict the future location of obstacles.
Lindqvist said: "Because NMPC works by predicting and optimizing the future state, we can also incorporate direct consideration of moving/dynamic obstacles by predicting the future position of moving/dynamic obstacles based on some measurement results." The method provides an elegant solution that integrates control, local path planning and dynamic obstacle avoidance functions in a single control layer."
Researchers have evaluated their NMPC protocol in many laboratory experiments. It is worth noting that their model can prevent collisions in various situations where the drone is surrounded by multiple moving obstacles.
Lindqvist said: "In the robotics and AI team at Luleå University of Technology, we attach great importance to the experimental verification of the theory." "In our recent work, we have shown how the NMPC architecture can even work with very fast moving obstacles. Down can also provide a collision-free trajectory without violating the required safety distance. I believe that avoiding obstacles is an interesting way to use drones in urban environments or to interact closely with humans."
The study emphasized the great potential of NMPC optimization schemes in enhancing the navigation capabilities of UAVs. The findings collected by Lindqvist and his colleagues can also inspire other research teams to use similar optimization techniques for robot control and path planning. Ultimately, this will help the development of drones and other mobile robots that can move safely in crowded and dynamic environments.
Lindqvist added: “As part of the CoSTAR group DARPA Underground Challenge, I believe that in the next year or so, we will invest a lot of energy to explore the topic of underground exploration and path planning for drones.” For the dynamic obstacle avoidance shown in this article, we plan to conduct future research aimed at integrating in-vehicle depth cameras (or similar devices) to track obstacles, and to improve and promote our obstacle prediction methods.
Avoid obstacle Spraying drone for agriculture
FD-10 avoid obstacles drone sprayer
Size: 1152*1152*630(Unfold State)
666.4*666.4*630(Fold State)
Tank: 10L
Weight: 11.6kg
Max take off weight:27kg
Flight time: 10-12 minutes(10kg payload)
Control distance: 3KM
Work efficience: 1.5 acres/flight
Function:
1. Foldable frame, easy to transport
2. Intelligent flight with autopilot
3. Intelligent flight memory, record break-point
4. Adjustable spray flow rate
5. Adjustable altitude and fly speed
6. Fail-safe, auto fly when out of control
7. Low voltage warning and auto return to base
8. Empty tank warning and auto return to base
9. Terrain following with MMW radar
10. Software(ground station and manage platform)
11. Obstacles avoidance
12. Double GPS
13. Multi-way charger
14. Intelligent 14000mAh battery